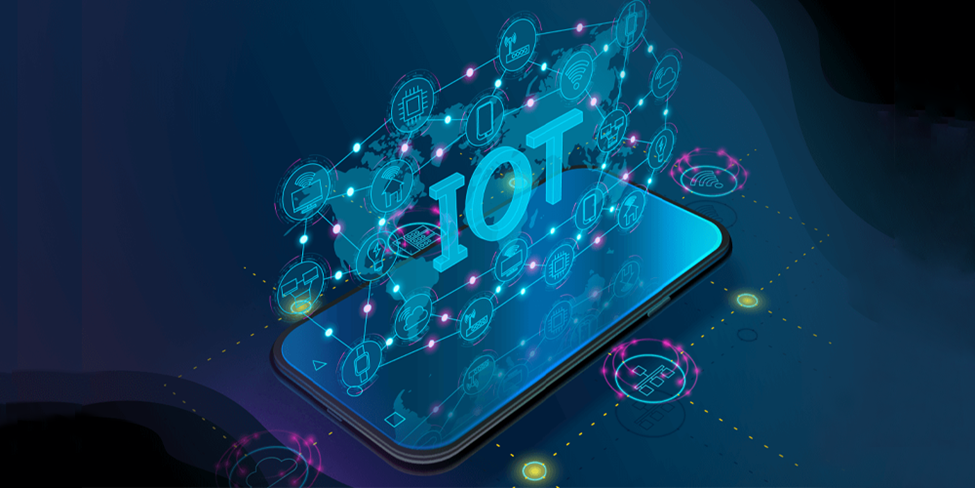
Introduction
The Internet of Things (IoT) has transformed the way we live and work, creating a vast network of interconnected devices that communicate and share data with each other. As the IoT continues to expand, so too does the need for more advanced technology to optimize its performance. One such technology is generative artificial intelligence (AI), which has the potential to significantly enhance the IoT and its applications. In this article, we will explore how generative AI can help the IoT, discuss the tradeoffs and challenges involved, and consider the broader implications for decision-makers. We will also delve into the technological details and end with a futuristic outlook on generative AI and IoT in 2030.
Generative AI and IoT: A Perfect Pairing
Generative AI refers to a class of machine learning algorithms capable of generating new data samples based on existing data. These models can learn patterns and features from the input data and create novel outputs that mimic the original data. In the context of IoT, generative AI can be used to improve processes, predict equipment failure, optimize energy consumption, and enhance security.
Generative AI models, such as Variational Autoencoders (VAEs) and Generative Adversarial Networks (GANs), have demonstrated their capabilities in various domains, including image synthesis, natural language processing, and drug discovery. By leveraging these powerful models, the IoT ecosystem can greatly benefit from their ability to generate valuable insights and make data-driven decisions.
Key Factors Impacting Generative AI in IoT
1. AI and Edge Computing: Edge computing involves processing data at the source, rather than sending it to a central server or the cloud. By integrating AI algorithms directly into IoT devices, edge computing can reduce latency, save bandwidth, and enhance security. Generative AI can be applied at the edge to make real-time decisions and predictions, improving IoT efficiency and responsiveness. One example is deploying lightweight GANs on IoT devices to perform tasks like image synthesis and anomaly detection.
2. IoT and Industrial IoT (IIoT): The IIoT refers to the use of IoT technology in industrial settings, such as manufacturing, transportation, and logistics. Generative AI can be particularly valuable in these contexts, as it can help predict equipment failure and optimize maintenance schedules, reducing downtime and increasing productivity. For instance, VAEs can be used to model the normal behavior of machinery and identify deviations, signaling potential issues before they escalate.
3. Cloud Computing: As IoT devices generate massive amounts of data, cloud computing can provide the necessary storage and processing power. The cloud can also host generative AI models, enabling them to analyze data from multiple devices and make more informed decisions. Cloud-based AI platforms, like Google’s TensorFlow and Amazon’s SageMaker, can facilitate the deployment and management of generative AI models for IoT applications.
Tradeoffs and Challenges
1. Balancing Local and Cloud Processing: Integrating generative AI into the IoT requires striking a balance between edge computing and cloud computing. While edge computing can provide real-time insights, it may not have the resources to handle complex AI models. On the other hand, relying solely on the cloud can lead to latency issues and increased bandwidth consumption. A hybrid approach, combining edge and cloud computing, can help address these concerns by distributing the computational load and optimizing resource allocation.
2. Security and Privacy Concerns: As IoT devices become more intelligent and interconnected, the potential for security breaches and privacy violations increases. Implementing generative AI in the IoT must be done carefully to ensure data integrity and protect sensitive information. Techniques such as federated learning and differential privacy can help mitigate these risks by enabling collaborative learning across devices without exposing sensitive data.
3. Model Complexity and Scalability: Generative AI models can be resource-intensive, which may limit their applicability in some IoT settings. Additionally, as the IoT continues to grow, the scalability of generative AI models will be a crucial factor in their success. Researchers are actively working on developing lightweight AI models, such as TinyGAN and MobileNets, that can be efficiently deployed on resource-constrained IoT devices without sacrificing performance.
4. Data Quality and Bias: Generative AI models rely on large amounts of data to learn patterns and generate meaningful outputs. Ensuring the quality and diversity of the data used to train these models is crucial to avoid biased or inaccurate results. IoT data can be noisy, incomplete, or unbalanced, making it challenging for generative AI models to capture the underlying patterns. Data preprocessing techniques, such as data cleaning, feature engineering, and data augmentation, can help improve data quality and ensure more accurate model outputs.
5. Interoperability and Standardization: As the IoT ecosystem becomes increasingly complex, ensuring seamless communication and interoperability between devices and systems is critical. Adopting standardized protocols and data formats can facilitate the integration of generative AI models into diverse IoT environments. Organizations like the Open Connectivity Foundation (OCF) and the Industrial Internet Consortium (IIC) are working towards establishing standards and best practices for IoT interoperability, including the use of AI technologies.
Generative AI and IoT in 2030
Looking ahead to 2030, the convergence of generative AI and IoT is poised to transform industries and our daily lives in profound ways. As IoT devices become more ubiquitous and intelligent, the role of generative AI will be essential in managing the complexity and scale of interconnected systems.
We can expect the IoT to become more proactive and autonomous, with generative AI models predicting and addressing issues before they arise, optimizing resource allocation, and enhancing overall system efficiency. Smart cities, powered by IoT and generative AI, will optimize traffic flow, reduce energy consumption, and improve public safety. In healthcare, wearable devices and remote monitoring systems will leverage generative AI to provide personalized health recommendations and predict potential health issues.
Moreover, the IIoT will see significant advancements in automation, with generative AI models driving predictive maintenance, optimizing supply chains, and enabling flexible manufacturing. In agriculture, IoT sensors and generative AI will enable precision farming, maximizing crop yields and minimizing resource waste.
However, the successful integration of generative AI into the IoT will require ongoing research and development, addressing challenges such as security, privacy, model complexity, and data quality. As we move towards this future, collaboration between industry, academia, and policymakers will be crucial in developing robust, scalable, and secure generative AI solutions for the IoT ecosystem.
Conclusion
The transformative potential of generative AI in the IoT landscape is nothing short of groundbreaking. By successfully integrating these two technological powerhouses, we can unlock unprecedented opportunities for innovation, efficiency, and sustainability across various industries and aspects of our daily lives. As we approach 2030, it is crucial that decision-makers, researchers, and industry professionals recognize the challenges and opportunities that lie ahead.
In this pursuit, addressing key issues such as security, privacy, model complexity, and data quality will be of paramount importance. By fostering interdisciplinary collaboration, adopting standardized protocols, and continuously refining generative AI models, we can create an IoT ecosystem that is not only intelligent but also scalable, secure, and robust.
The vision of a future powered by generative AI and the IoT is one where our cities are more sustainable and responsive, industries are more efficient and agile, and individual lives are more connected and empowered. This bold vision is not an unattainable dream; it is a tangible reality within our reach, provided we make thoughtful and informed decisions today.
As you embark on the journey to harness the power of generative AI and IoT, Zigron stands as your trusted partner, ready to help you develop cutting-edge solutions and achieve your visions. With our expertise in AI, IoT, and advanced technologies, Zigron bridges the gap between your goals and the realization of innovative, efficient, and secure applications. Our team of dedicated professionals will work closely with you to unlock the full potential of generative AI and IoT, transforming your ideas into reality. Don’t wait any longer – take the first step towards a smarter, interconnected future by contacting us at https://www.linkedin.com/in/zigron/ or sales@zigron.com.